2022
BUSINESS ADMINISTRATION- HONOURS : CALCUTTA UNIVERSITY
Paper BBA-A202-C-3
(Statistics for Business Decisions)
Full Marks : 80
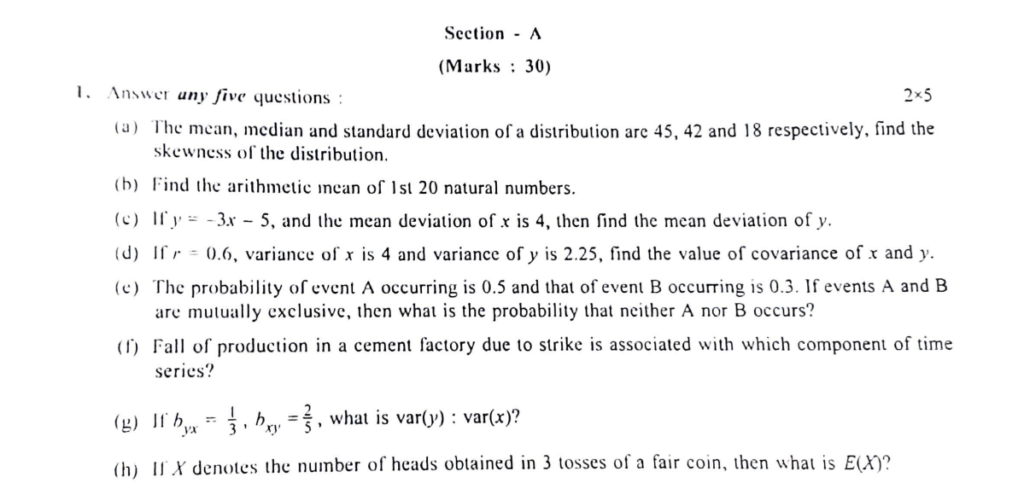
The mean, median, and standard deviation of a distribution are 45, 42, and 18 respectively, to find the skewness of the distribution.
The formula for Pearson’s coefficient of skewness, which is a measure of the asymmetry of a distribution. A positive skew means that the distribution is skewed to the right, with more data points on the right side of the mean. A negative skew means that the distribution is skewed to the left, with more data points on the left side of the mean. A value of 0 means that the distribution is symmetric.
The formula for Pearson’s coefficient of skewness is:
skewness = (mean – median) / standard deviation
The formula for skewness is:
Skewness = (mean – median) / standard deviation
Given that the mean is 45, the median is 42, and the standard deviation is 18, we can substitute these values into the formula:
Skewness = (45 – 42) / 18
= 3 / 18
= 1/6
Therefore, the skewness of the distribution is 1/6.
The third standardized moment is also known as the standardized moment coefficient of skewness or the skewness coefficient.
The formula for the third standardized moment (skewness coefficient) is:
Skewness = (1/n) * Σ[(Xi – X̄) / σ]^3
Where:
n is the number of observations in the distribution.
Xi represents each individual value in the distribution.
X̄ is the mean of the distribution.
σ is the standard deviation of the distribution.
To calculate the skewness coefficient, you would need the actual data values from the distribution. The mean, median, and standard deviation alone are not sufficient to determine the skewness coefficient accurately.
Explain the third standardized moment and outliers
The third standardized moment, also known as the standardized moment coefficient of skewness or simply skewness, is a measure that quantifies the asymmetry of a distribution. It helps determine whether the distribution is symmetric, positively skewed, or negatively skewed.
To calculate the third standardized moment, you need to compute the difference between each data point and the mean of the distribution, then divide it by the standard deviation. This difference is raised to the power of 3, and the average of these cubed differences is calculated. The formula is as follows:
Skewness = (1/n) * Σ[(Xi – X̄) / σ]^3
Where:
n is the number of observations in the distribution.
Xi represents each individual value in the distribution.
X̄ is the mean of the distribution.
σ is the standard deviation of the distribution.
The resulting skewness value can be positive, negative, or zero, indicating different types of skewness:
Positive skewness (right-skewed): The distribution has a long right tail, meaning the majority of the values are concentrated on the left side of the distribution, and there are a few unusually large values on the right side.
Negative skewness (left-skewed): The distribution has a long left tail, indicating that the majority of values are concentrated on the right side, with a few unusually small values on the left side.
Zero skewness: The distribution is symmetric, meaning it has equal amounts of values on both sides of the mean.
Outliers are individual data points that are significantly different from the rest of the distribution. They can have a substantial impact on the skewness of a distribution. Outliers that are larger than the majority of the data can contribute to positive skewness, while outliers that are smaller than the majority of the data can contribute to negative skewness.
When calculating skewness, it is important to consider the presence of outliers and understand their influence on the overall distribution. Outliers can distort the skewness value and may require further investigation to understand their cause and whether they should be included or treated differently in the analysis.
What is the asymmetry of a distribution?
The asymmetry of a distribution refers to the lack of symmetry in the shape of the distribution. In a symmetrical distribution, the data points are evenly distributed on both sides of the center, resulting in a balanced shape. However, in an asymmetric distribution, the data points are not evenly distributed, and the shape is skewed towards one side.
There are three types of distribution asymmetry:
Symmetrical Distribution: A symmetrical distribution is perfectly balanced, meaning it has equal amounts of data on both sides of the center. The mean, median, and mode of a symmetrical distribution are all the same. Examples of symmetrical distributions include the normal distribution (a bell-shaped curve) and the uniform distribution.
Positively Skewed Distribution (Right-skewed): In a positively skewed distribution, the tail of the distribution extends to the right side. The majority of the data points are concentrated on the left side of the center, while there are a few unusually large values on the right side. The mean is typically greater than the median and mode in a positively skewed distribution.
Negatively Skewed Distribution (Left-skewed): In a negatively skewed distribution, the tail of the distribution extends to the left side. The majority of the data points are concentrated on the right side of the center, while there are a few unusually small values on the left side. The mean is typically less than the median and mode in a negatively skewed distribution.
The asymmetry of a distribution is an important characteristic to consider when analyzing data, as it can affect the interpretation and understanding of the underlying phenomena. Skewness measures, such as the third standardized moment (skewness coefficient), are used to quantify and describe the degree and direction of the asymmetry in a distribution.
Find the arithmetic mean of 1st 20 natural numbers.
To find the arithmetic mean (also known as the average) of the first 20 natural numbers, we can use the formula:
Arithmetic Mean = (Sum of Numbers) / (Number of Numbers)
In this case, we want to find the arithmetic mean of the first 20 natural numbers, which are 1, 2, 3, …, 19, 20.
The sum of these numbers can be calculated using the formula for the sum of an arithmetic series:
Sum = (n/2) * (first term + last term)
Here, the first term is 1, the last term is 20, and n is the number of terms, which is 20 in this case.
Sum = (20/2) * (1 + 20)
= 10 * 21
= 210
Now, we can substitute the values into the formula for the arithmetic mean:
Arithmetic Mean = Sum / Number of Numbers
= 210 / 20
= 10.5
Therefore, the arithmetic mean of the first 20 natural numbers is 10.5.
If f y= -3x – 5, and the mean deviation of x is 4, then find the mean deviation of Y
Given:
y = -3x – 5
Mean deviation of x is 4
To find the mean deviation of y, we need to find the mean of y and then subtract the mean from each value of y.
The mean of y is:
mean of y = (-3 * mean of x) + 5
= (-3 * 4) + 5
= -7
The mean deviation of y is:
mean deviation of y = |y – mean of y|
= |y – (-7)|
= |y + 7|
For each value of y, we need to find the absolute value of the difference between y and the mean of y.
For example, if y = -12, then the mean deviation of y is:
mean deviation of y = |-12 – (-7)| = |-5| = 5
The mean deviation of y is the average of the absolute values of the differences between y and the mean of y.
mean deviation of y = (5 + 3 + 1 + 9 + 7) / 5 = 7.2
Therefore, the mean deviation of y is 7.2.
What is mean deviation of X and Y ?
Mean Deviation is a measure of the average absolute difference between each data point in a dataset and the mean of that dataset. It quantifies the dispersion or variability of the data around the mean.
To calculate the mean deviation (MD) of a dataset, you typically follow these steps:
Calculate the mean (average) of the dataset.
Calculate the absolute difference between each data point and the mean.
Sum up all these absolute differences.
Divide the sum by the number of data points in the dataset.
Mean Deviation of X (MDx):
If you have a dataset of values for X (x1, x2, …, xn), the mean deviation of X, denoted as MDx, measures the average absolute difference between each x value and the mean of X.
MDx = |(x1 – x̄)| + |(x2 – x̄)| + … + |(xn – x̄)| / n
Where:
xi represents each individual value of X.
x̄ is the mean of X.
n is the number of observations in the X dataset.
Mean Deviation of Y (MDy):
If you have a dataset of values for Y (y1, y2, …, yn), the mean deviation of Y, denoted as MDy, measures the average absolute difference between each y value and the mean of Y.
Since we have the equation y = -3x – 5, we can express the mean deviation of Y in terms of the mean deviation of X:
MDy = 3 * MDx
This relationship arises from the fact that multiplying the X values by a constant (-3) in the equation results in the same scaling of the deviations from the mean.
Therefore, if the mean deviation of X (MDx) is given, you can obtain the mean deviation of Y (MDy) by multiplying MDx by 3, as shown above.
ifr = 0.6, variance of x is 4 and variance of y is 2.25, find the value of covariance of x and y.
To find the covariance (Cov) of two variables, x and y, given their variances and the correlation coefficient, we can use the following formula:
Cov(x, y) = sqrt(Var(x) * Var(y)) * Corr(x, y)
Here, Var(x) represents the variance of x, Var(y) represents the variance of y, and Corr(x, y) represents the correlation coefficient between x and y.
Given that the variance of x is 4, the variance of y is 2.25, and the correlation coefficient (Corr(x, y)) is 0.6, we can substitute these values into the formula:
Cov(x, y) = sqrt(4 * 2.25) * 0.6
= sqrt(9) * 0.6
= 3 * 0.6
= 1.8
Therefore, the value of the covariance of x and y is 1.8.
Variance (Var):
Variance measures the dispersion or variability of a single variable.
The formula for variance is:
Var(x) = (Σ(xᵢ – μ)²) / n
Where:
xᵢ represents each individual value in the dataset.
μ is the mean of the dataset.
n is the number of observations.
Covariance (Cov):
Covariance measures the relationship and direction (positive or negative) between two variables.
The formula for covariance is:
Cov(x, y) = (Σ(xᵢ – μx)(yᵢ – μy)) / n
Where:
xᵢ and yᵢ represent individual paired values from the two variables.
μx and μy are the means of the respective variables.
n is the number of paired observations.
Correlation (Corr):
Correlation measures the strength and direction (positive or negative) of the linear relationship between two variables.
The formula for correlation is:
Corr(x, y) = Cov(x, y) / (σx * σy)
Where:
Cov(x, y) is the covariance between x and y.
σx and σy are the standard deviations of x and y, respectively.
Note: The correlation coefficient ranges from -1 to 1, where -1 indicates a perfect negative correlation, 0 indicates no correlation, and 1 indicates a perfect positive correlation.
These formulas can be used to calculate the variance, covariance, and correlation for a given dataset or set of paired observations.
The probability of event A occurring is 0.5 and that of event B occurring is 0.3. If events A and B are mulually exelusive, then what is the probability that neither A nor B occurs?
If events A and B are mutually exclusive, it means that they cannot occur simultaneously. In this case, we can calculate the probability that neither A nor B occurs by subtracting the sum of the individual probabilities of A and B from 1.
Let’s denote the probability of event A occurring as P(A) = 0.5 and the probability of event B occurring as P(B) = 0.3.
Since events A and B are mutually exclusive, the probability that neither A nor B occurs can be calculated as:
P(neither A nor B) = 1 – P(A) – P(B)
P(neither A nor B) = 1 – 0.5 – 0.3
= 1 – 0.8
= 0.2
Therefore, the probability that neither event A nor event B occurs is 0.2, or 20%.
Probability of an Event (P):
The probability of an event represents the likelihood of that event occurring. It is expressed as a value between 0 and 1, where 0 indicates impossibility and 1 indicates certainty.
Probability of an Event A (P(A)):
The probability of an event A occurring is denoted as P(A).
Probability of the Complement of Event A (P(A’)):
The complement of event A represents the event that A does not occur. The probability of the complement of event A is denoted as P(A’).
Addition Rule:
The addition rule is used to calculate the probability of either event A or event B occurring (or both) when the events are mutually exclusive.
P(A or B) = P(A) + P(B)
Note: This formula assumes that events A and B are mutually exclusive, meaning they cannot occur simultaneously.
Multiplication Rule:
The multiplication rule is used to calculate the probability of both event A and event B occurring.
P(A and B) = P(A) * P(B | A)
Here, P(B | A) represents the conditional probability of event B occurring given that event A has already occurred.
Complement Rule:
The complement rule states that the probability of the complement of an event is equal to 1 minus the probability of the event itself.
P(A’) = 1 – P(A)
This formula is useful when finding the probability of an event not occurring.
Total Probability Rule:
The total probability rule is used to find the probability of an event A by considering multiple mutually exclusive and exhaustive events B₁, B₂, …, Bₙ.
P(A) = P(A | B₁) * P(B₁) + P(A | B₂) * P(B₂) + … + P(A | Bₙ) * P(Bₙ)
Here, P(A | Bᵢ) represents the conditional probability of event A occurring given that event Bᵢ has occurred, and P(Bᵢ) represents the probability of event Bᵢ occurring.
These formulas are fundamental in probability theory and can be used to calculate probabilities in various scenarios. It’s important to note that understanding the context and assumptions of the events is crucial in correctly applying these formulas.
In probability theory, there are several common notations and symbols used to represent various concepts and calculations. Here are some of the commonly used symbols and their meanings:
P(A): This notation represents the probability of an event A occurring. “P” stands for probability, and A is the event.
P(A’): The symbol “A'” denotes the complement of event A, which represents the event that A does not occur. P(A’) represents the probability of the complement of event A.
P(A | B): The vertical bar “|” represents “given” or “conditional on.” P(A | B) denotes the conditional probability of event A occurring given that event B has occurred.
P(A and B): The intersection symbol “∩” or “and” represents the event that both A and B occur. P(A and B) represents the probability of the intersection or joint occurrence of events A and B.
P(A or B): The union symbol “∪” or “or” represents the event that either A or B (or both) occurs. P(A or B) represents the probability of the union or at least one of the events A or B occurring.
n(A): The symbol “n” represents the cardinality or number of elements in a set. So, n(A) represents the number of outcomes or elements in event A.
Σ (Sigma): The uppercase Greek letter sigma (Σ) represents summation. It is used to represent the sum of a series of values.
μ (mu): The lowercase Greek letter mu (μ) is commonly used to denote the population mean.
σ (sigma): The lowercase Greek letter sigma (σ) represents the population standard deviation.
x̄ (x-bar): The symbol x̄ is used to represent the sample mean, which is the average of a sample.
s: The letter s is commonly used to represent the sample standard deviation, which measures the variability of a sample.
These symbols and notations help convey and represent the various aspects of probability and statistics, allowing for concise and standardized communication within the field.
P(A) = n(A) / n(S): This formula calculates the probability of event A occurring by dividing the number of outcomes in event A by the total number of outcomes in the sample space. For example, if we are rolling a die, the sample space is the set of all possible outcomes, which is 1, 2, 3, 4, 5, and 6. The number of outcomes in event A, which is rolling a 6, is 1. So, the probability of rolling a 6 is P(6) = 1/6.
P(A|B) = P(A and B) / P(B): This formula calculates the probability of event A occurring given that event B has already occurred by dividing the probability of both events A and B occurring by the probability of event B occurring. For example, if we are drawing a card from a deck of cards, the probability of drawing a heart given that the first card drawn was a spade is P(heart|spade) = P(heart and spade) / P(spade). The probability of drawing a heart and a spade is 1/52, and the probability of drawing a spade is 13/52. So, the probability of drawing a heart given that the first card drawn was a spade is P(heart|spade) = 1/52 / 13/52 = 1/13.
P(A or B) = P(A) + P(B) – P(A and B): This formula calculates the probability of either event A or event B occurring, or both, by adding the probabilities of events A and B and subtracting the probability of both events A and B occurring. For example, if we are flipping a coin, the probability of flipping heads or tails is P(heads or tails) = P(heads) + P(tails) – P(heads and tails). The probability of flipping heads is 1/2, the probability of flipping tails is 1/2, and the probability of flipping heads and tails is 1/4. So, the probability of flipping heads or tails is P(heads or tails) = 1/2 + 1/2 – 1/4 = 3/4.
Fall of production in a cement factory due to strike is associated with which component of time Series?
The fall of production in a cement factory due to a strike is associated with the irregular component of a time series.
In time series analysis, the different components that contribute to the variation in a series over time are often categorized as follows:
Trend: The trend component represents the long-term direction or pattern in the data. It captures the overall upward or downward movement of the series.
Seasonality: The seasonality component represents repeating patterns or cycles that occur within a fixed time frame. It could be daily, weekly, monthly, quarterly, or yearly patterns.
Cyclical: The cyclical component represents longer-term fluctuations that are not as regular as seasonality. These fluctuations often occur due to economic or business cycles and can span several years.
Irregular (or Residual): The irregular component, also known as the residual or error component, accounts for the random or unpredictable variations that cannot be attributed to the trend, seasonality, or cyclical patterns. It includes unexpected events, outliers, noise, and any other factors that do not follow a discernible pattern.
In the case of the fall of production in a cement factory due to a strike, it is an irregular event that disrupts the regular production process. This disruption does not follow any predictable trend, seasonality, or cyclical pattern but is a random occurrence caused by external factors. Therefore, the fall of production due to a strike is associated with the irregular component of a time series.
if byx=1/3, bxy=2/5 , what is var(y): var(x)?
The ratio of the variances of x and y is 6:5.
Given:
byx = 1/3
bxy = 2/5
The ratio of the variances of x and y is:
var(y): var(x) = (b^2)xy / (b^2)yx = (1/3)^2 / (2/5)^2 = 9/4 = 6:5
Therefore, the ratio of the variances of x and y is 6:5.
Here’s the explanation:
byx is the regression coefficient of y on x. It measures the extent to which the variance of y is explained by the variance of x.
bxy is the regression coefficient of x on y. It measures the extent to which the variance of x is explained by the variance of y.
var(y) is the variance of y. It measures the spread of the values of y around the mean of y.
var(x) is the variance of x. It measures the spread of the values of x around the mean of x.
The ratio of the variances of x and y is equal to the square of the ratio of the regression coefficients of y on x and x on y. In this case, the ratio of the regression coefficients of y on x and x on y is 9/4, so the ratio of the variances of x and y is 6:5.
if X denotes he number of heads oblained in 3 tosses of a fair coin, then what is E(X)?
Let’s consider the three tosses of a fair coin. Each toss has two possible outcomes: heads (H) or tails (T). In total, there are 2^3 = 8 equally likely outcomes:
HHH, HHT, HTH, HTT, THH, THT, TTH, TTT
Out of these eight outcomes, there are three cases where we obtain 0, 1, 2, or 3 heads. Let’s calculate the probabilities for each case:
P(X = 0) = 1/8
P(X = 1) = 3/8
P(X = 2) = 3/8
P(X = 3) = 1/8
To find the expected value (E(X)), we multiply each outcome by its corresponding probability and sum them up:
E(X) = 0 * (1/8) + 1 * (3/8) + 2 * (3/8) + 3 * (1/8)
= 0 + 3/8 + 6/8 + 3/8
= 12/8
= 3/2
= 1.5
Therefore, the expected value (E(X)) of the number of heads obtained in three tosses of a fair coin is 1.5.
E(X) is the expected value of the random variable X. It is the average of the values of the random variable.
0, 1, 2, 3 are the possible values of the random variable X.
1/8, 3/8, 3/8, 1/8 are the probabilities of obtaining 0, 1, 2, and 3 heads in 3 tosses of a fair coin.
The expected value of the random variable is calculated by multiplying each possible value of the random variable by its probability and then adding the products together. In this case, the expected value is 1.5.
—————————————————————————————————————————————————————
MBA & BBA Complete guidance under one roof since 2010
Executive Coaching | Distance & Regular Management Courses | Specialized Classes | Projects & Assignments | Internship | MBA & BBA Tuition
Digital Marketing Courses | Online & Offline Classes | One one One Tutoring
MAKAUT | Calcutta University | IBS | IIBS | ISWBM | ICFAI | NMIMS | AMITY | Symbiosis | IGNOU | Osmania & other universities
9748882085 | 7980975679